Thinking a lot about data storytelling, hiring, and early-stage infrastructure? We are too. Grab some time here and let’s chat.
If you ask ten founders or early-stage operators when and why they made their first data hire, you’ll find that it was not because they reached a specific milestone (e.g., crossing $X in ARR or Xk users). Instead, it happens when the founding team realizes that their understanding of their own business narrative is not as deep as they need it to be.
As you read above (or maybe you attended our first dinner earlier this week!), I’m working with a lot of early analytics hires these days. Specifically, I’m interested in how folks wade through the messy data they inherit and quickly spin up the infrastructure they need so they can start delivering narratives and insights to their team, customers, and investors. That “wading” process, turns out, is quite painful. And most startups end up kicking it down the road.
The problem, we’ve found, is not analyzing the data. We can bang our heads against Excel for a couple hours and figure it out. Instead, the problem is in gathering, formatting, and automating the data. We’ve talked to a lot of startups in the past couple of months, and found that we were repeating ourselves fairly often:
You do NOT need a full-blown state-of-the-art data infrastructure. At your stage, it’s expensive overkill.
Here’s a classic transportation analogy:
You probably are currently walking/crawling with one-off Excel analyses. They get the high-level job done, but not very well and definitely not very quickly.
“State-of-the-art” data infra is a BMW convertible: Data warehouse, transformations, a heavy BI dashboard, etc. It’s expensive and requires a lot of maintenance. You’re a startup and we do not recommend this for you.
We need to get you a mountain bike (or, depending on your business, one of those fancy new electric Citi Bikes). A mountain bike is versatile, flexible, and can take a beating.
What does this “mountain bike” actually look like? It means a strong understanding of your data model (what data are you storing?), a set of simple SQL queries to easily export and manipulate this data (automate Excel), and finally, a well-instrumented set of front-end events that help you understand your user journey (maybe analyzed through Amplitude et al). For some, it also may be a reverse ETL to send relevant data into your marketing or operations tools so your team can feel like superhumans.
How many of you know you are leaving insights on the table because you lack simple data infrastructure? Let us know.
Happy weekend,
Raman at Rhetoric
📚 What’s made me a better storyteller this week
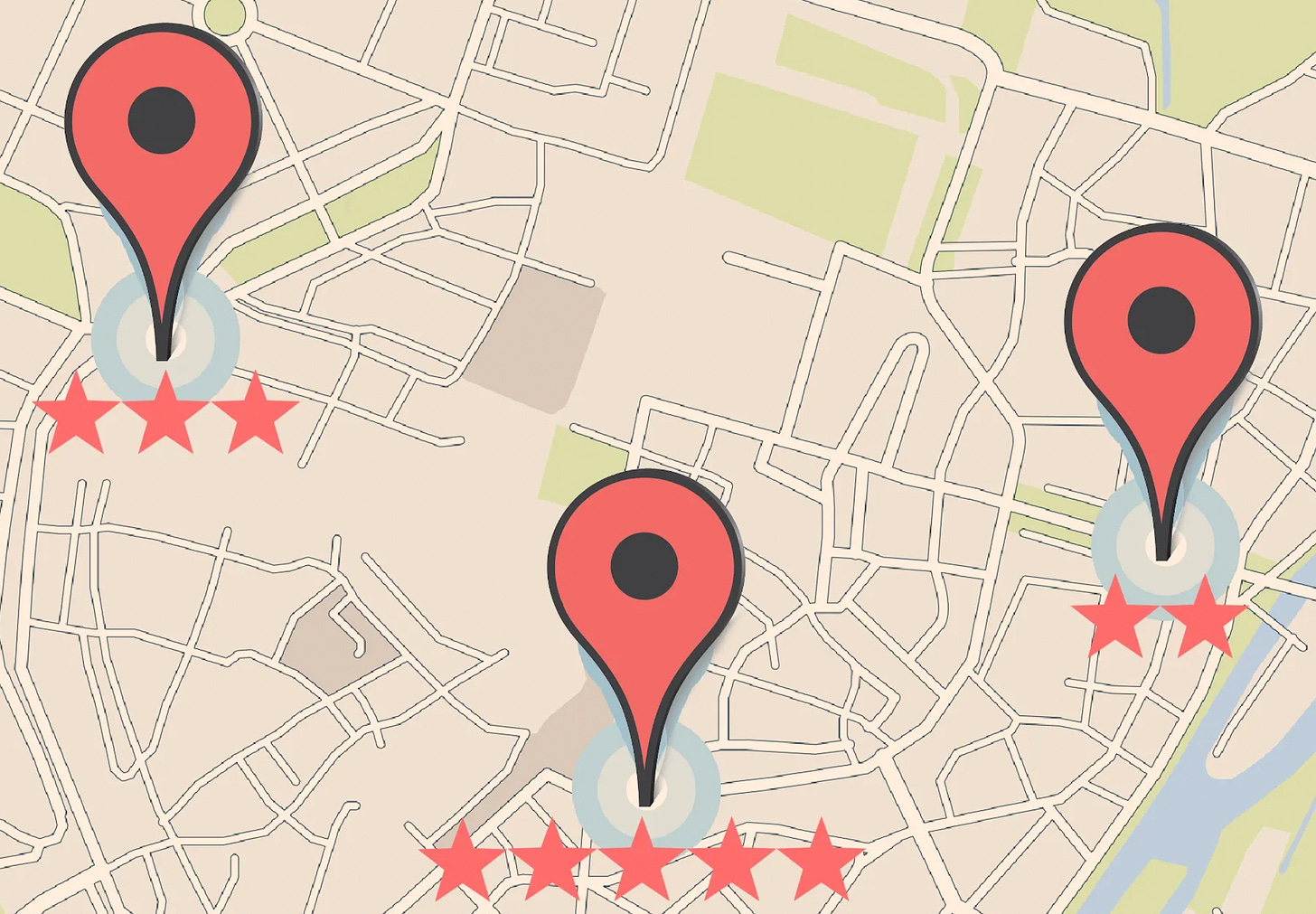
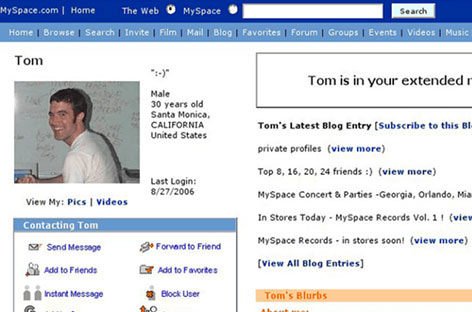
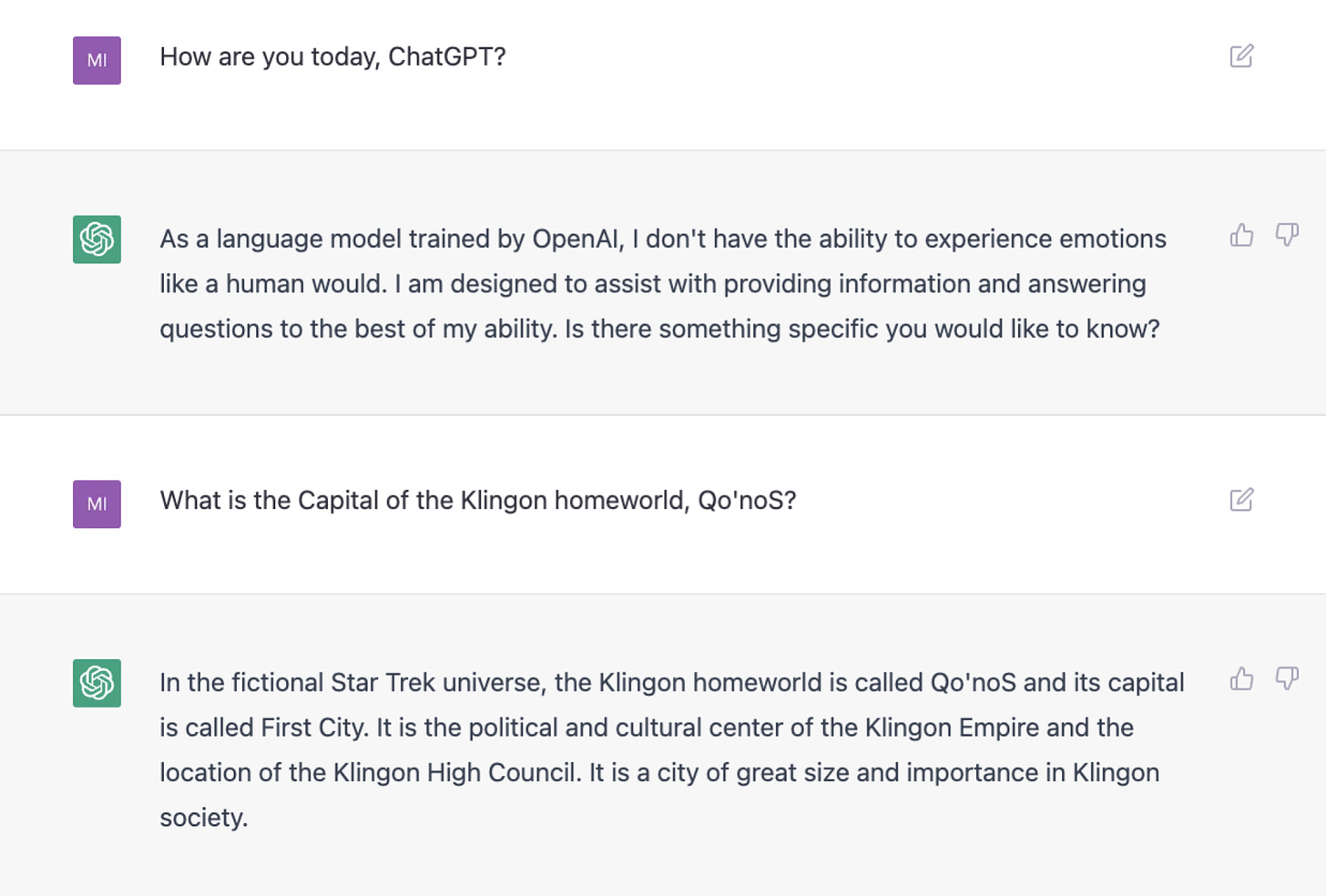